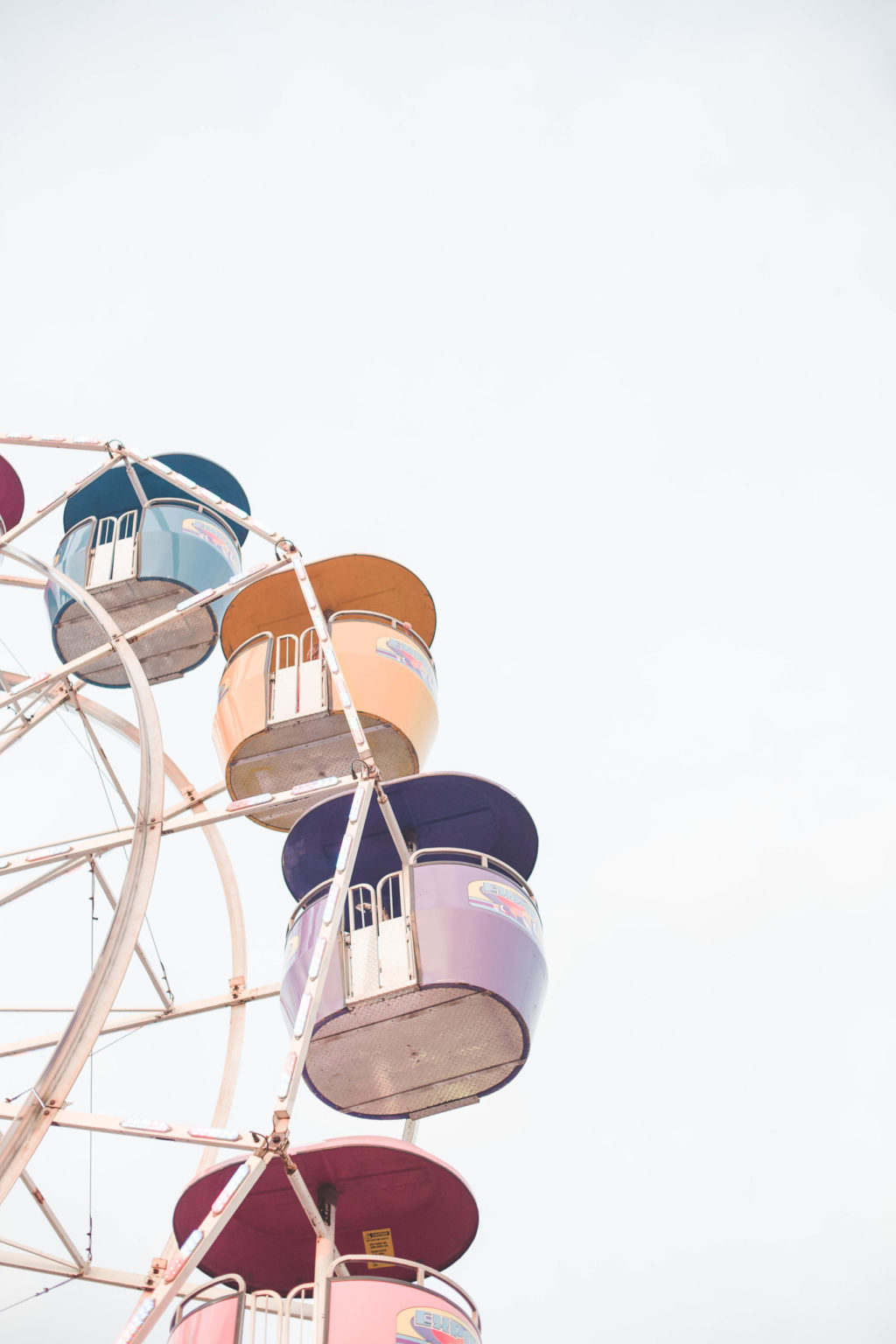
Predictive Audiences vs. Lookalike Models: Overview & Use Cases
What steps can you take to make this year the best yet for your marketing team?
Surely achieving key goals like increased engagement, loyalty and revenue are top of mind. And while there are many steps you can take to reach these goals, one of the best places to start is making better use of the data and tools already at your disposal.
For example, how can you activate the rich behavioral data you already have about your customer base? How can you use it to not only engage with shoppers on your website, but also through targeted email campaigns?
Your answer lies in the power of predictive audiences.
What is audience modeling?
Audience modeling includes applications of predictive intelligence to determine audiences based on a use-case. This could include factors such as:
- Discount affinity
- Channel affinity
- Lifecycle stage
- Purchase activity
- Likelihood to convert
- Likelihood to unsubscribe
How Predictive Audiences Work
Predictive audiences use artificial intelligence to learn about your customers, their product preferences and their spending habits. As more data becomes available over time, the technology powering these audiences can make predictions based on more subtle and granular signals.
Predictive audiences are so powerful because they allow you to proactively engage customers in a personalized way using leading indicators that help you predict their future activity. This opens up a world of possibilities by allowing your retail marketing team to proactively prime customers to take certain actions, instead of reacting to actions they have already taken.
Even though predictive analytics offer a wealth of benefits to retailers, many teams still build audiences based on historical data, with the most common tactic being lookalike models. Unfortunately, models that rely on historical data alone fall woefully short when compared to predictive audiences. That’s because past behavior alone does not necessarily indicate future behavior. Let’s take a closer look at how these two forms of audience modeling stack up.
How Do Predictive Audiences Differ from Lookalike Models?
Predictive audiences and lookalike models may seem to have a lot in common at first. Both are forms of audience modeling that rely on data to find and segment new customer audiences. However, the differences between predictive audiences and lookalike models are what ultimately defines them.
Lookalike models help businesses determine which audiences are similar to previous successful target audiences to drive more conversions. They use first-party data to outline the demographics, psychographics, preferred channels, etc. of audiences that tend to engage with a certain campaign and find customers that closely match that description by compiling large datasets. From there, marketers can add these lookalike audiences to existing campaign workflows to expand their reach. However, it takes a long time to collect the amount of first-party data required to create lookalike models – so it’s not the most efficient way of finding new audiences. Additionally, it uses only historical data, which not only assumes that customers will continue the same behavior over time, but also limits retailers’ ability to facilitate product discovery and encourage new behaviors.
Predictive audiences solve these problems by building audiences that determine a customer’s propensity to take a certain action in the future. They also expand on the use cases of lookalike models by allowing your marketing team to build audiences based on factors like predicted affinities for products, predicted lifetime value, predicted lifecycle stage and even predicted discount affinity.
For example, an AI-driven solution can predict a customer’s next action (e.g. making a purchase within the next week, unsubscribing from emails) or new interests (e.g. product categories) based on leading indicators like recent activity onsite and over email. In turn, this will unveil a new audience based on their likelihood to take a certain action.
Knowing what a shopper will do next or is more interested in with a high degree of certainty is a boon for retailers when it comes to personalizing the shopping experience and proactively engaging shoppers before they turn to the competition. The best way to do this is to adopt a retail-specific predictive marketing solution that can natively understand key types of data, such as browsing and purchase behavior, to help your team build highly personalized campaigns around predictive audiences.
6 Use Cases for Predictive Audiences
After understanding the power of predictive analytics, you may have a few questions. What types of predictive audiences can you build? And how should you put them to work to boost the performance of your marketing campaigns? Start with these six initiatives:
1) Increase relevance with targeted product recommendations
As consumers’ standards for retail experiences continue to heighten, so does the importance of relevant marketing. One of the best ways to achieve this goal is to surface more targeted recommendations for customers at scale by building audiences based on predicted product and category affinities.
When it comes to these personalized product recommendations, two key use cases stand out:
- Expanding the reach of already targeted campaigns: If you’re running a targeted campaign to promote a specific product or category, who do you include in your audience? If you’re like most retailers, you go after past purchasers and recent browsers. But what if you could expand that audience without sacrificing relevance? Layering in an audience based on predicted affinity allows you to do just that by identifying customers who are likely interested in the products featured but have not yet demonstrated that interest. This approach has allowed retailers to increase the size of their audience by upwards of 60x, increase open rates by 77% and increase revenue per email by over 700%.
- Scaling back on full file batch emails: Batch and blast might be here to stay, but what a batch email looks like is most certainly changing. Going forward, the most successful batch emails will go to fewer people while pulling in more revenue, and the key to making this happen lies in sending those emails to the right people. Specifically, building an audience based on predicted affinity can help you scale back on send volume by targeting only customers with a demonstrated interest or predicted affinity for the products featured in each email. This approach helped one retailer increase campaign revenue by more than 3x, even while reducing the send volume by seven million.
2) Get smarter about lifecycle marketing
Paying attention to the lifecycle stage of your customers can make a big difference. Because active, at-risk, lost and non-buyers engage with your brand differently, marketing to each group in a distinct way can help boost engagement and drive more purchases.
But using static time periods to determine their stage in the customer journey (e.g. customers who haven’t made a purchase in 90 days are at-risk and customers who haven’t made a purchase in 120 days are lost) is flawed, because each customer has his/her own unique buying cadence. What’s normal buying behavior for one shopper might be a red flag for another shopper. That’s where predictive customer lifecycle models come into play by placing shoppers into lifecycle stages based on their individual buying cadences.
One of the biggest benefits that comes from building audiences based on predicted lifecycle stages is the ability to create personalized messaging that sends to customers right when they deviate from their buying cycle to become at-risk. Too often, retail marketers miss their opportunity to save at-risk customers because they’ve waited too long and those customers have already moved on to another brand. On the flip side, many retailers send come back messaging too early and put off still-loyal shoppers. Building an audience of at-risk customers based on predicted lifecycle stage and then setting up an automated “come back” trigger that sends to customers who have deviated from their individual buying cadence can help you take steps to save those shoppers at just the right time, every time.
3) Spend your paid marketing dollars wisely
Let’s face it: Paid marketing on channels like Facebook and search is expensive, and the competition (and therefore the costs) are only increasing. While there’s nothing you can do to decrease those costs, you can make sure you spend your digital advertising dollars wisely to increase your return on investment.
One of the best ways to do so is to build an audience based on predicted customer lifetime value, that way you can focus more of your ad dollars on your highest value customers. For one retailer, this approach led to a 56% increase in ROI on media buys.
In this case, building an audience based on predicted customer lifetime value proves more fruitful than building an audience based on historical lifetime value because past purchases alone don’t indicate what someone is likely to spend in the future. In contrast, predicted lifetime value takes into account more than just past spend by evaluating signals like frequency of site visits and browsing history to identify the customers that will be most valuable to your brand in the long run.
4) Foster loyalty with intelligent replenishment offers
In today’s world, convenience counts for a lot. And if you can present your customers with a reminder to replenish items at the exact time they need to restock (plus an easy way to follow through on that reminder), that’s the ultimate convenient — not to mention relevant — retail experience.
Achieving this goal might sound difficult, but it’s actually well within reach. Once again, the key to success lies in a predictive audience. In the case of replenishment, a predictive model can make it easy for your marketing team to send customers personalized reminders based on their individual buying cadences by:
- Identifying products within your catalog that customers purchase in a replenishable manner
- Tracking customers’ individual buying cadences for those replenishable products
From there, your team can set up an automated replenishment trigger that proactively notifies customers to restock products they previously bought at just the right time, presenting them with an offer that’s hard to refuse.
5) Minimize unsubscribes based on channel preferences
Did you know that every unsubscribe costs retailers anywhere from $18 to $36? At scale, that means retailers lose hundreds of thousands of dollars in revenue every year to unsubscribes. While some unsubscribes are necessary to a healthy email marketing program, there’s a lot you can do to minimize your unsubscribe rate.
To start, you might use a predictive model that evaluates customers’ likelihood to take certain actions like open, click and unsubscribe from emails. In this case, you want to build an audience of customers who have a high likelihood to unsubscribe from emails and begin excluding that audience from upcoming promotional emails. Ideally, you can nurture those customers on other channels until they’ve warmed up to the idea of receiving emails from you once more and are no longer at risk of unsubscribing.
6) Preserve your margins (and reputation) on full price buyers
While some customers will hardly ever buy anything at full price, others have no sticker shock. Furthermore, many of those full price buyers may be turned off by sale offers if they come to associate your brand with heavy discounts.
Building an audience based on predicted discount affinity can help you balance these two types of customers. Let’s say you have an upcoming sale or want to promote products that recently decreased in price. Rather than notifying all of your customers or even just those with a predicted affinity toward the relevant products, you can also layer in discount affinity to make sure those messages only go to customers flagged as discount shoppers. By removing your full price buyers from the distribution list, you not only preserve your margins, but you also preserve your reputation with shoppers who don’t want to associate with what they consider to be discount brands.
How Do Leading Retailers Use Predictive Audiences?
How do leading retailers put these types of predictive audiences to work to boost the performance of their marketing campaigns? Check out our Bluecore Marketing Lookbook for a sampling of marketing emails that span everything from behavioral and merchandising triggers to batch emails and lifecycle outreach.
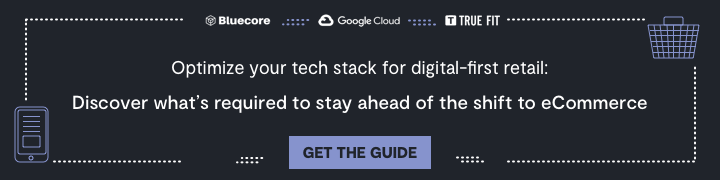