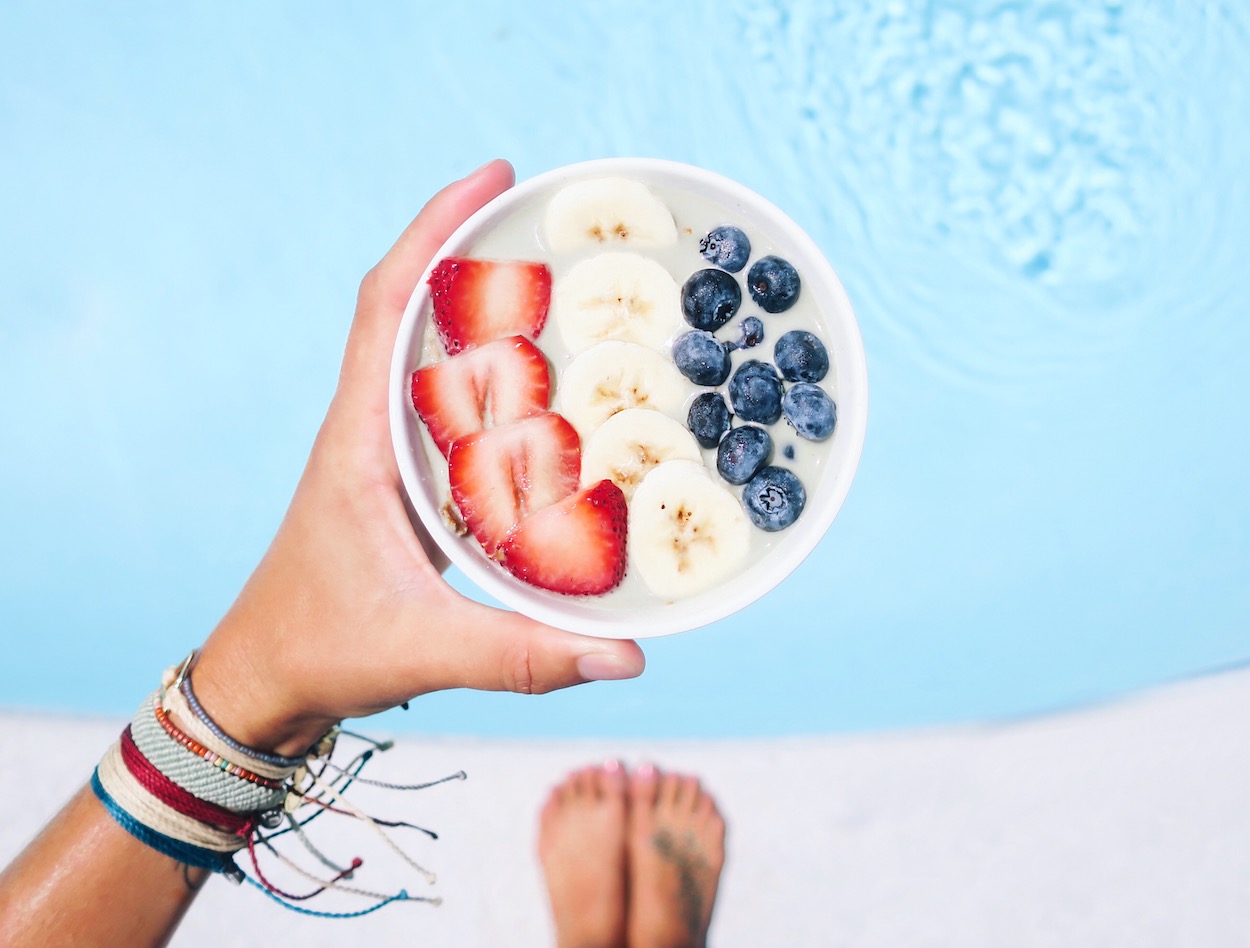
Pulling Back the Curtain on the Value of Predictive Technology
For all the value that predictive technology offers, the models behind it remain shrouded in mystery for many retail marketing teams.
When retail marketing teams first approach predictive analytics, some of the biggest questions that regularly surface center around the data that goes into the model. For example, how much data do you need to make accurate predictions? And based on that, how long do you need to wait until you start seeing any value from the models? Demystifying these questions empowers marketers to confidently use AI-driven predictive technology and reaffirms the need to get started with predictive tools sooner rather than later.
What is Predictive Marketing?
Predictive marketing is a set of processes and practices that uses data to forecast a customer’s future behavior. For instance, teams often use predictive marketing to discover which products a customer has an affinity for, allowing you to craft a marketing strategy that capitalizes on their growing interests. Or you can use it to calculate a customer’s predicted lifetime value, helping your team focus on customers that will drive the most revenue long term.
As your team continues to feed the predictive models more high-quality customer, behavior and product data, your predictions will become even more precise. Over time, you’ll gain a strong understanding of which customers to target, when to target them and what they need to hear.
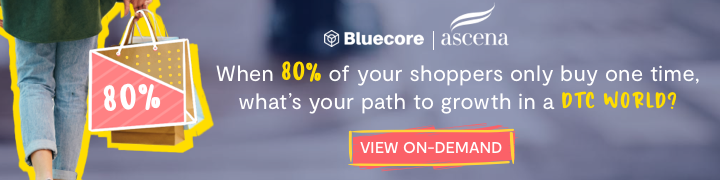
The Growing Value of Predictive Marketing Technology
With predictive modeling, the more marketing data you have, the more powerful the model gets. These models can provide value from the get-go, but that value will increase over time as they have more and more data on which to base their predictions. Additionally, some predictions (like category affinity) require less variety and quantity of data than others (like predicted lifetime value and lifecycle stage) to ramp up.
One quick way to get a lot of value out of predictive models from day one is to upload historical purchase data. While the models will become more robust as view/search/cart and other data accumulates, purchase history alone can get things going right off the bat. Since most retailers have collected mountains of order data for years now, feeding this data into the system will boost the model performance from day one. With historical purchase data, the models will start to learn patterns of things such as purchase frequencies, order sizes and popular combinations of products.
The models will continue to learn a lot more about customers and products as they acquire more marketing data with which to work, such as product views and category searches. Over time, the technology will learn more and more about each customer and their product preferences and spending habits as well as the relationships and patterns of interactions between customers and products.
How Predictive Marketing Works
One way to think about how the amount and variety of data can impact the power of predictive technology is to compare the models’ outputs to a photograph.
When you first get started with predictive technology, you’ll likely only have a month or so worth of data in the system. At this point, it might be like looking at a blurry photograph.
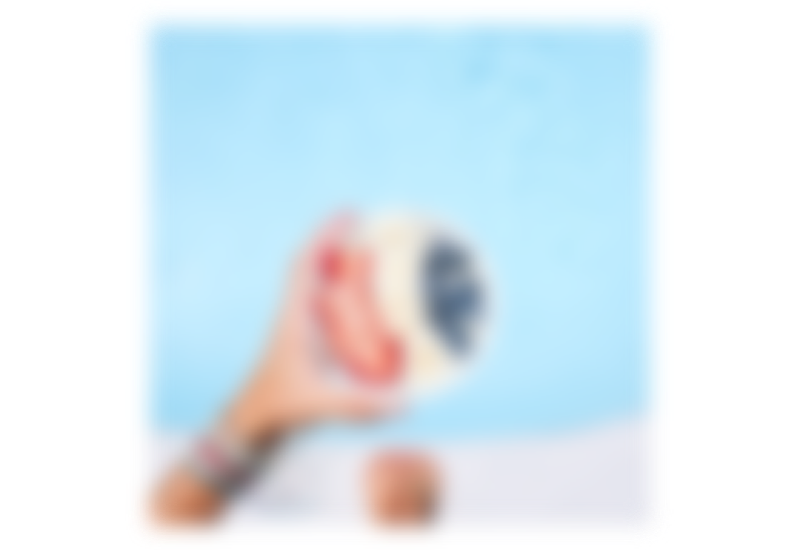
You can still get value out of the system because it gives you something to narrow in on, but you can only go so deep. For example, you might be able to look at predicted customer lifetime value, but to start you’ll only be able to segment audiences into pretty broad buckets, such as the top 50% versus the bottom 50%. That’s because the model does not yet have enough information to confidently produce more targeted results.
As you collect more data over time, the image will start to become clearer and you’ll be able to zoom in a bit closer. For instance, once you have a quarter’s worth of data, you might be able to zoom in to the point where you can look at the top 25% of customers based on predicted lifetime value and even overlay their affinity for discounts or various product categories.
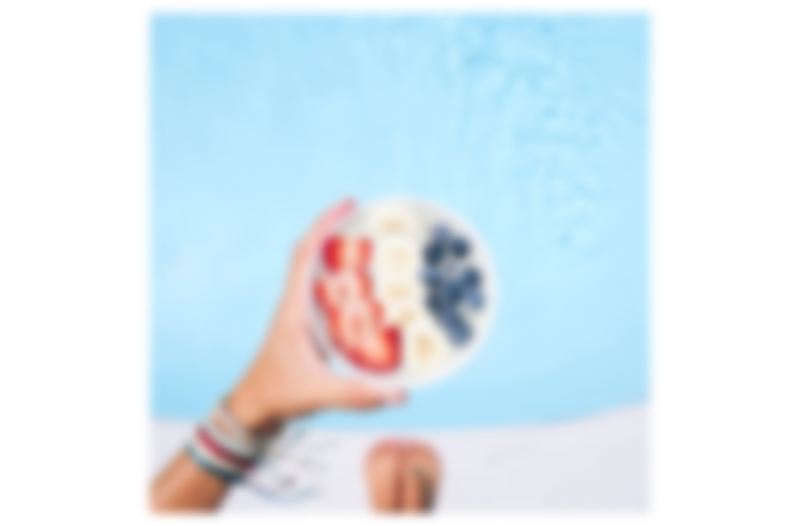
With six months of data you begin to get a pretty crisp picture, but two year’s worth of data brings it to another level because that allows you to capture seasonal behaviors and year-over-year patterns. With that amount of marketing data, you’ll have a much clearer image that allows you to zoom in on narrow segments, such as the top 5% of customers based on predicted lifetime value as well as their affinity to a new product line, all segmented by their likelihood to convert on email (of course this is just one example of many).
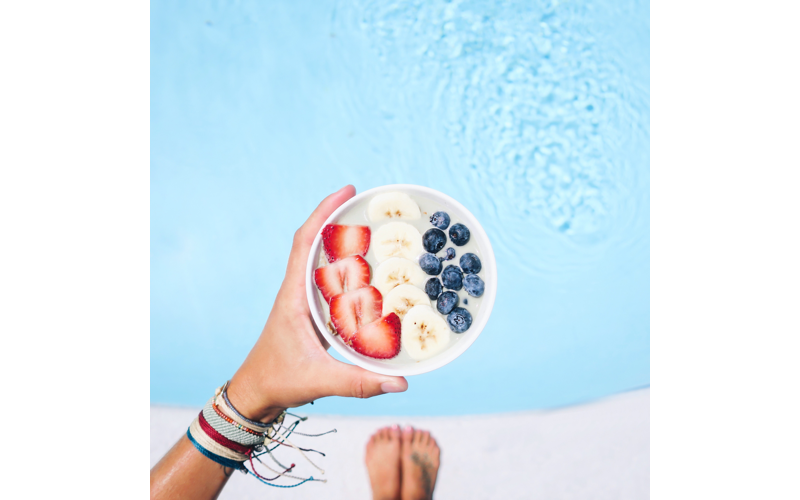
So when all is said and done, predictive technology can provide value from the get go, but that value will significantly increase over time as data accumulates. You can still make useful predictions with a small amount of data, but you can only make those predictions on very strong signals. As the data in your system grows, you can begin to make predictions on more subtle and granular things.
3 Compelling Use Cases for Predictive Marketing Technology
Predictive marketing technology has a seemingly endless number of potential use cases. For now, let’s go over three use cases that directly translate to greater ROI for retailers.
1. Predict Customer Churn
While growing a customer base is incredibly important, keeping the customers you already have is arguably more important to a retailer’s success. New customers cost more to acquire, whether it be an investment in advertising or anything else to attract those shoppers and educate them about your brand. If your team only focuses on generating new customers and doesn’t strive to build customer loyalty, you will be left constantly treading water and never experience growth.
So what if you could tell who is about to stop buying from your brand? Having the ability to predict customers who are at-risk allows marketers to engage with key audience segments and retain critical long-term revenue. This allows your marketing team to save your best at-risk customers, count on more consistent revenue and create accurate forecasts.
2. Predict and Grow Customer Lifetime Value
Have you ever been prepared to purchase something at full price and then get pleasantly surprised to receive a discount code? You can thank suboptimal retail marketing processes for that. Every year, brands forfeit hundreds of thousands of dollars by awarding discounts to shoppers who would otherwise purchase items at full price. By understanding which of your customers would buy from you without a discount, your team can stop throwing away your margins.
Taking that one step further, your marketing team can also better understand discount shoppers in order to provide them with markdowns that mean something to them and will spark action. For example, a retailer may find it beneficial to offer discounts to customers only if they have a high predicted lifetime value and a high discount affinity, since these discounts will ensure the customer becomes more loyal and spends more on the brand. Then, the discount that they offer can differ depending on the customer’s unique identity – while some customers may need a 10% discount on their entire cart, others may be swayed with an offer for free tailoring services.
3. Predict Customer Actions
Perhaps the most valuable aspect of predictive data in email marketing is to anticipate customers actions in regard to how they will engage with your brand. Although there are certainly trends reporting how people of different ages and demographics prefer to receive communications from brands, customers are individuals with unique behaviors. Understanding an individual’s propensity to open, click or unsubscribe from an email, or engage with advertisements and push notifications, can mean the difference between them turning into a loyal customer or opting out of your brand’s communications forever. Predictive analytics can empower your team to identify these customers before the point of no return, fight email fatigue and engage customers in the channel that they most prefer.
An Example of How Predictive Technology Drives Results
What’s wrong with sticking to the traditional analysis of past behaviors alone? Nothing necessarily, but making marketing decisions based on analyzing past behaviors doesn’t provide anywhere near the results that making decisions with predictive technology can. To better understand the value that this technology can provide, let’s look at some results from marketing campaigns built using predictive category affinity models.
Consider the case of one major furniture retailer that decided to test the value of predictive technology by sending the same category promotion email to its full email list split into two groups:
- A small but targeted audience comprised of customers who had previously browsed the category and customers with a high predicted affinity toward the category
- Everyone else in the email database
The first, targeted email went to 90k customers, which was one million fewer customers than received the second, batch and blast email, but the first email generated double the orders and 19% more in revenue.
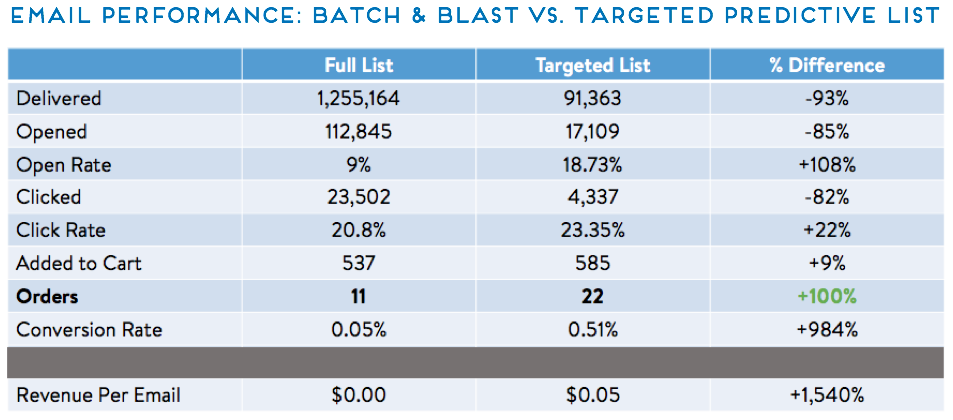
The same performance pattern holds true even when looking at past behavior versus predicted affinity. Specifically, Steve Madden also tested the value of predictive technology, this time by sending the same category promotion email to an audience of past buyers and an audience with a predicted affinity toward the category. Much to the team’s surprise, the predictive audience was 60x bigger than the audience of past buyers and 100% of the campaign revenue came from the predictive audience.
And the value of predictive technology extends across channels. For instance, one footwear and apparel retailer saw a 2.5x increase in site conversions by doing onsite personalization for category affinity audiences, while one technology retailer increased its return on advertising spend from 3.5x to 11x when prospecting on Facebook using an audience with a predicted affinity toward a specific category and a high predicted lifetime value.
Why is Predictive Marketing Technology So Valuable?
What is it that makes predictive technology so powerful? There are three particular reasons why predictive technology delivers results like those detailed above:
Traditional analysis is only part of the story
Basing your analysis solely on past behavior can cause you to miss certain opportunities because it doesn’t account for all of the complicated relationships between the various behaviors, which means you have to know what you’re looking for. As a result, it’s harder to get the big picture and you might miss out on important details. It’s kind of like taking a very zoomed in view of a photograph. That view can tell you a lot, but without the bigger picture alongside it, you might be missing out. Predictive technology helps surface that bigger picture.
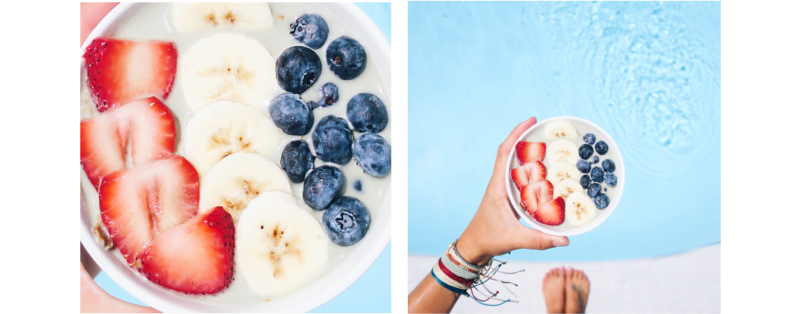
Improve the reach of personalized campaigns
Adding predictive insights, such as category affinity, to your campaign can help broaden the reach of your efforts without sacrificing personalization. It does this by expanding the size of audiences for different campaigns while maintaining a very targeted approach. This type of expansion has proven extremely profitable for several brands.
Engage proactively
Targeting customers based on these types of predictions can help you engage with them in a more proactive way rather than just reacting to what they do. Proactive engagement is extremely important at a time when customers are looking for highly personalized, curated experiences. In fact, as your predictive models get smarter over time, you can even use them to emulate the ultimate value-add retail experience — the small shop owner who knows her customers so well that she calls them when something comes in that she thinks they’ll like.
At the end of the day, predictive technology allows you to use the customer data you’re already collecting in a more meaningful way by making smarter decisions about who to target. Perhaps best of all, it makes the analysis and decisioning process easy by eliminating guesswork and manual inputs.
Get Started Sooner Rather Than Later
Given that predictive technology can provide a valuable boost to your marketing efforts and that it only gets more powerful over time as it collects more data, it’s important to get started with predictive technology sooner rather than later.
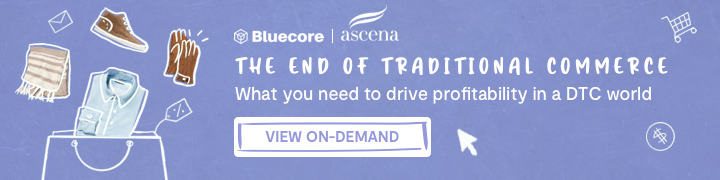