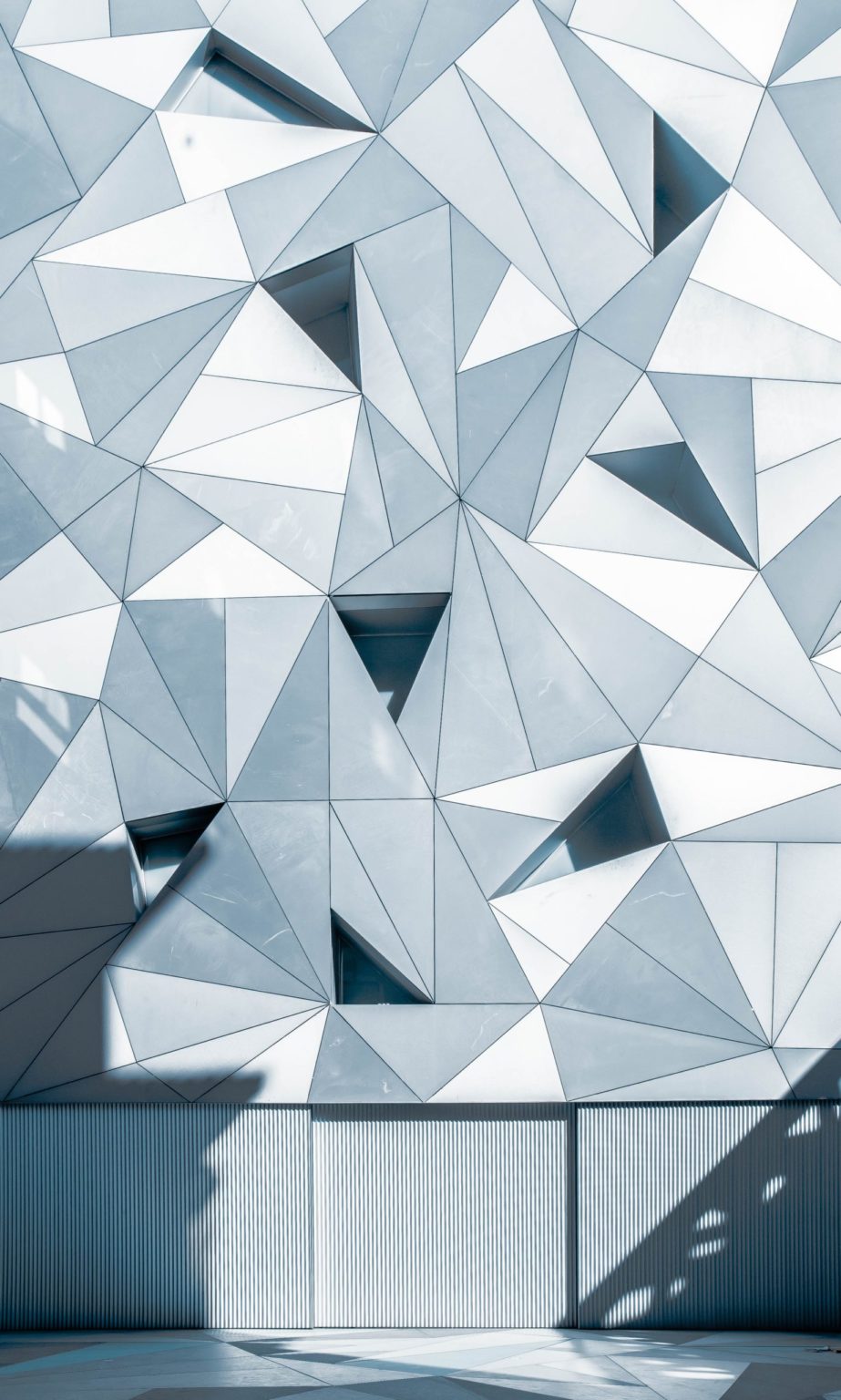
The Data Model Powering the Top Performing Retail Marketing Programs
Why retail marketing teams need three specific types of data and the data model that makes it possible
In a world of the Email Death Spiral, retailers everywhere are struggling to maintain the status quo — let alone improve performance. But to succeed in today’s competitive market, most brands acknowledge they need to figure out a way to increase campaign performance with less effort.
It sounds like a pipe dream, but for fast-growing retailers who care about personalization, the levers are at your fingertips. It starts with a technology purpose-built for retail, personalization and scale. And while there are many characteristics of the right technology that make this possible, the most critical is the underlying data model.
The Traditional Data Model Falls Short for Retail
Typically, brands have data stored across multiple systems: Those to manage identity, audience segmentation, product recommendations, execution and ecommerce are just some of the most common.
In search of a way to reduce the number of data feeds in play, many brands are now evaluating CDPs, which promise to become the new upstream data tool that manages anonymous and known identifiers in a single place.
But even modern CDPs miss an essential piece of the equation for retailers: Product data.
In these sense, product data is not just a product catalog; it’s the dynamic elements of how product metadata changes, such as when prices and inventory levels change. Today this data primarily gets pulled into marketing campaigns via merchandising, but going forward marketers who want to achieve true personalization will use this dynamic product data to connect people to products beyond what the merchandising team is pushing.
Retailers Need a System Built on Three Types of Data
What retailers need to deliver truly personalized experiences that drive performance — and do so with less effort — is a retail-specific data model that unifies behavior, customer and product data.
It’s this three-piece data model that allows retailers to understand the “when” (behavior), “who” (customer) and “what” (product) required to create differentiated and timely experiences for individual shoppers.
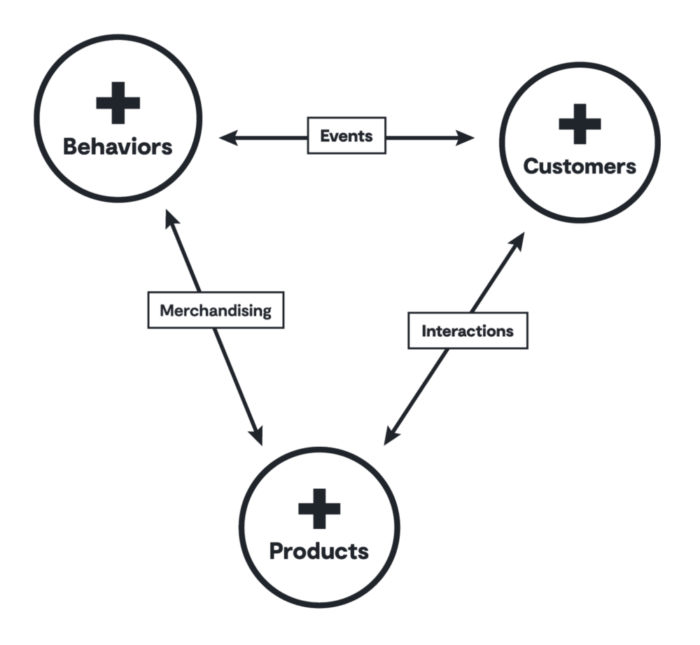
What a Retail-Specific, Three-Piece Data Model Makes Possible
How does a retail-specific, three-piece data model change the game for marketers?
What You Want to Do: Connect Shoppers to Relevant Products in a Moment of Need — at Scale
Let’s say you work at a health and beauty retailer and I’m a customer of yours who’s in the market for new skincare products. How would you find a specific shopper like me — along with two million others who are active on your list — and connect me to the right product?
To start, you would need to know what I’m searching for at the exact time when I’m searching for it. Beyond that, the more predictions you can layer in about me, the more targeted you can get.
Ideally, you want to show me a product recommendation based on (1) a new arrival (2) for someone with a high affinity for skin cream who hasn’t purchased yet and (3) has a high likelihood to buy full price products in the next three days.
How to Do It: Use a Retail-Specific, Three-Piece Data Model to Combine and Take Action on Data
The beauty of a retail-specific data model is that it unifies the core data elements you need to power relevant, individualized campaigns at scale (behavior, customer and product data). This unification allows you to query each edge of that three-piece model in real-time, starting with any intersection you want.
Let’s say you start at the intersection of product and product behavior to find new arrivals in stock.
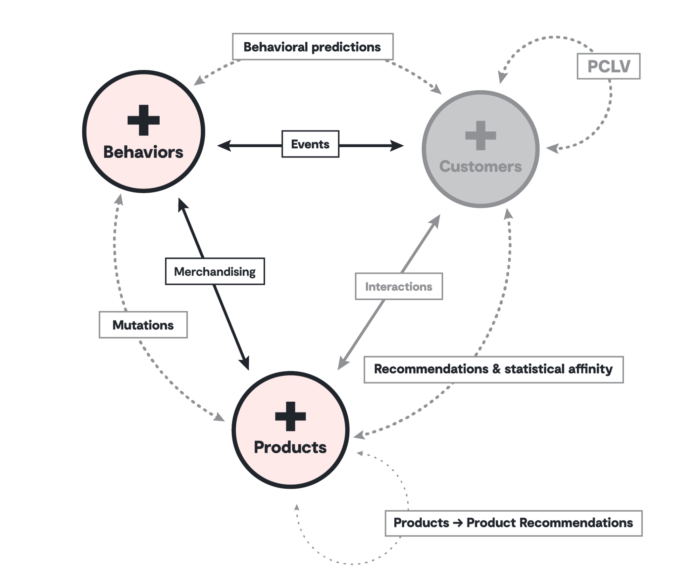
From there, you can easily overlay those new arrivals with your customer data and user level scoring that predicts category affinity to identify which of your customers will be most interested in the specified products.
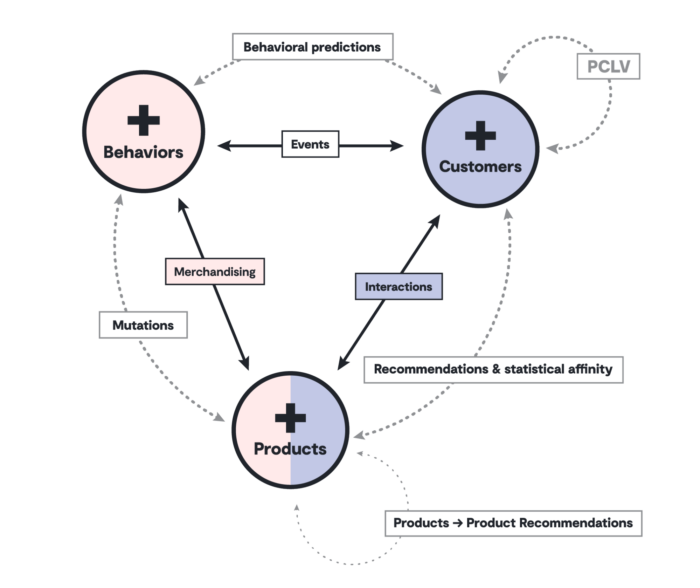
Lastly, you can narrow down the audience to those with a high likelihood to purchase in the next three days to ensure that your communication about the products hits shoppers in a moment of need.
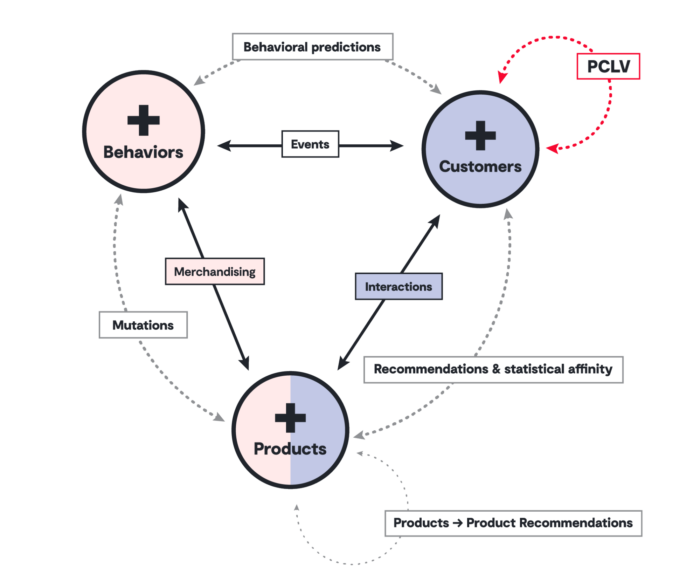
Critically, you can start with any intersection, meaning you can lead with a specific set of products (as in the example here) or a specific set of customers (based on interest in certain products or behavior at a certain time).
Why It’s Different: Proactively Deliver Individualized, Timely Recommendations at Scale
With a generic data model, there’s no way to understand the product catalog. As a result, affinity scoring is based only on past purchase behavior, which limits your exposure to customers who have previously bought the products in question. But this approach falls flat, because what you really want is to capture the much larger universe of customers with an affinity to purchase the products going forward.
A retail-specific, three-piece data model as described here not only captures that forward-looking view, but it also generates product recommendations at the individual customer level. This setup means that every single query you might make can scale across hundreds of thousands of individuals in your active list. And once you have that, you can deliver truly individualized communications at scale while staying aligned to business goals around revenue, retention and sell-through.
Supercharge Your Marketing with the Right Data Model
What else can a retail-specific, three-piece data model do for your marketing team? Once you have the right foundation in place, the opportunities to increase performance are plentiful.
Check out our eBook on retail’s email personalization problem to find out what it takes and learn more about why product data is so critical to success in retail.
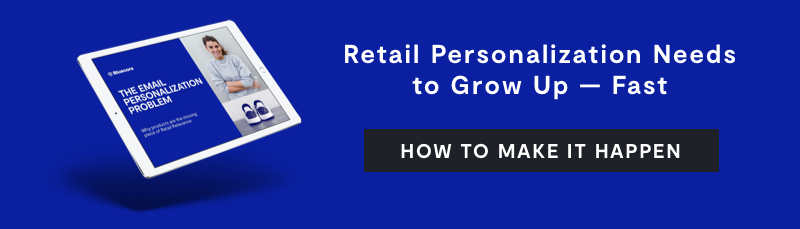