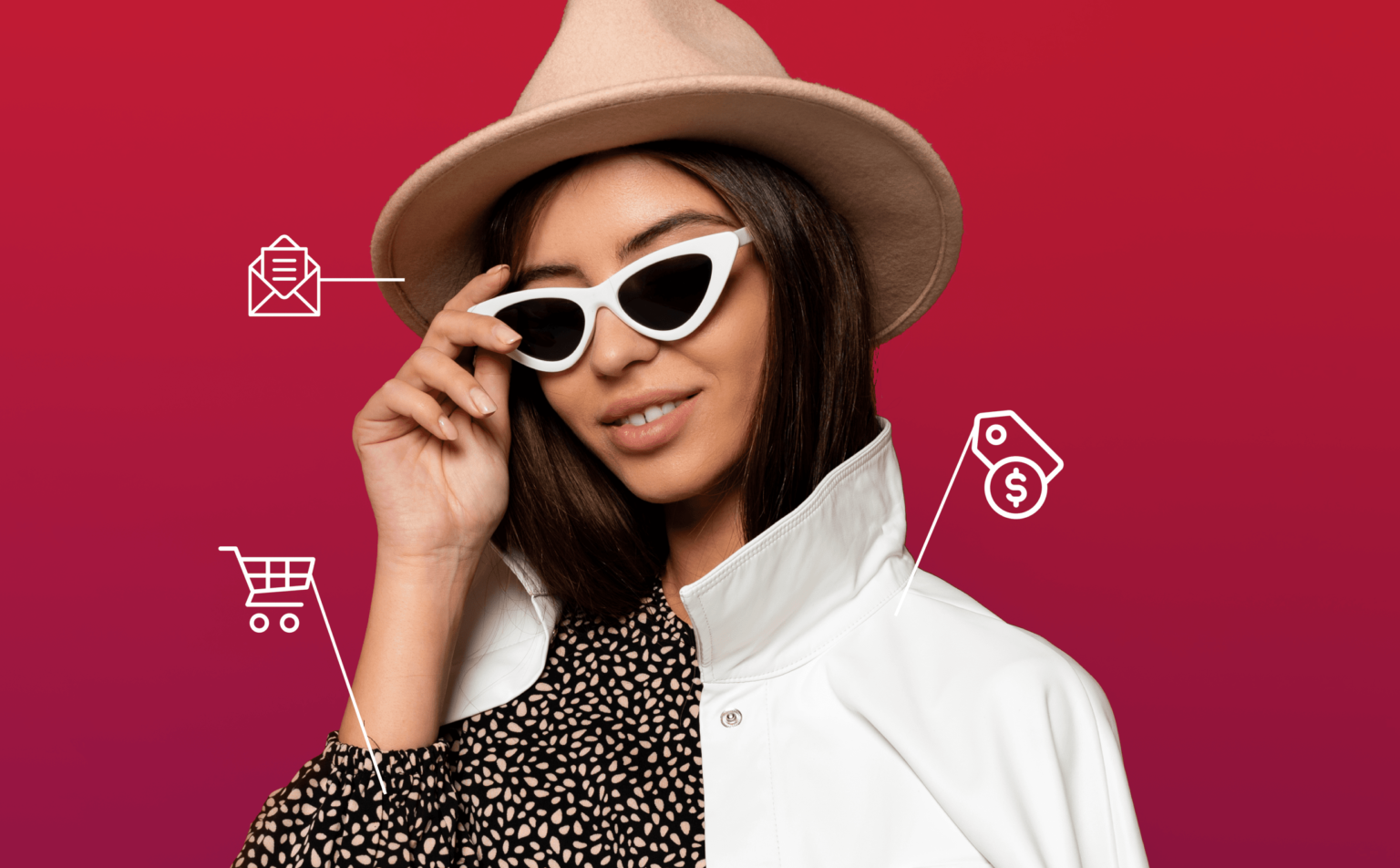
Optimize Paid Media Campaign Spend with AI-Powered Audiences
Paid media is a nearly $400 billion dollar industry worldwide. For retail, in particular, it offers enormous opportunities to reach new customers and re-engage existing ones. However, the reality of paid media — particularly following the rollercoaster of 2020 — is quite different.
Over the past several years, paid media has become highly competitive and increasingly expensive. This has put a strain on capital and operating budgets as brands aim to fund the seamless omnichannel experience. The accelerated shift to digital and reliance on these channels to generate qualified traffic and revenue due to COVID-19 only exacerbates the situation. And when you factor in rising customer acquisition costs and the death of the third party cookie, the promise of those opportunities seems far beyond reach.
As a result, we end up with something of a death spiral in which brands continue to throw larger budgets at paid media but see diminishing returns, which only makes the advertising industry even more expensive.
But it doesn’t have to be so grim. Bringing more intelligent, first party data into the process can restore the promise of paid media and deliver on key goals for your brand.
Bringing Intelligence to Paid Media with AI-Powered Audiences
The status quo of paid media for retailers looks something like this: Using a generic seed list of past 30 day purchasers or opted-in customers as a basis for lookalike audiences or setting up pixel-based audiences to retarget customers who visited the site in the last seven days.
On the one hand, this approach uses first party data, which is big positive. First party data is future proof: It will always be available because it comes from the direct relationship between your brand and your customers. This stands in stark contrast to third party data, which is subject to increasingly strict regulations.
On the other hand, this approach doesn’t offer any sort of intelligence to find new high value customers, targeting existing high value customers or even show them the products they’re most likely to buy. So while it may help source some new revenue, there’s no way to ensure that revenue comes from the “right” customers who will provide ongoing value for your brand. In fact, it’s often a fast (and expensive) path to accelerating retail’s already high one-and-done buyer problem.
Instead, what your team needs is a way to pair this first party data with intelligence in the form of dynamic AI-powered audiences, for example predictive models that can layer in additional context like predicted lifetime value, predicted product or category preference, predicted likelihood to buy and predicted discount affinity. Best of all is a solution that will automatically update those lists as shoppers take new action on your eCommerce site.
This approach solves the challenges of the status quo by making it possible to find the “right” customers: Those who are most likely to be loyal to your brand and are ready to buy at a given point in time. It also helps your brand connect shoppers to products they love and are therefore most likely to buy.
While there’s nothing your brand can do about the cost of paid media, taking this more intelligent approach optimizes that spend by ensuring you allocate it toward the most high value efforts. As a result, this intelligence can increase your brand’s return on ad spend (RoAS) by up to 130%.
4 Ways to Bring AI-Powered Audiences to Paid Media to Support Key Retail Goals
Diving deeper, how does bringing this intelligence to your paid media efforts support key retail goals around acquisition and retention? And what does doing so entail? Here are four outcomes this approach can power:
Acquire New High Value Customers
Acquire new high value customers at a lower cost by only targeting customers who have a high likelihood to shop with your brand and are similar to your existing customers who are predicted to spend the most with your brand in the next two years.
How: Instead of uploading a static seed list of customers based on factors like email opted-in, loyalty status or 30 day purchase activity to power lookalike capabilities, use predictive models to build a list of highly valuable customers based on factors like predicted lifetime value, spend level, category interests and/or purchase frequency.
Solve the One-and-Done Buyer Problem
Become more exacting in the strategies your brand uses to get the all-important second purchase and increase customer lifetime value as a result by specifically targeting recent one-time buyers with relevant recommendations on paid media.
How: The targeting available through paid media platforms doesn’t provide granular customer data, so getting this list typically requires working with internal analytics teams to build and upload that information in batches. However, that data can quickly become stale as customers make purchases. A platform that can collect full customer 360 data, including customer behavior data like online and offline purchases as well as product data, gives your marketing team access to build audiences to resolve those challenges. With that setup, your team can build an audience of first-time buyers and set up a daily sync to ensure the data is always based on the most recent customer activity.
Increase Customer Loyalty and Lifetime Value
Augment your retargeting strategy to drive more qualified traffic to your eCommerce site by engaging customers with more relevant recommendations at the time when they are most likely to buy.
How: Retargeting customers based on their most recent browsing behavior misses the complete picture of how these shoppers have engaged with your brand over time. In contrast, if your team can target customers with more context than just their last action on your eCommerce site, such as the products they’re most likely to be interested in, their likelihood to convert at a given point in time or their lifecycle status (e.g. active or at-risk), you can run more relevant campaigns. This relevance extends to everything from the products featured in the ads to the timing of the ads, and when you get that mix right, you should be able to ensure you reach customers when they are most likely to buy with the products they are most likely to purchase — thereby improving the customer experience and driving more repeat purchases.
Scale and Coordinate Personalization Across Channels
Enable your team to scale and coordinate personalized cross-channel strategies within one audience targeting tool. Giving your marketing team direct access to audience building tools also enables them to become very nimble and react to changes in customer demand for specific products or categories.
How: Traditionally, access to data that provides context for paid media campaigns, such as customer behaviors, CRM data or predictive audiences, is limited. Many brands have turned to CDPs for help, but this can lead to resource-intensive, highly manual processes that make it difficult to scale in a meaningful way. Using a more marketer-friendly tool that aligns merchandising and marketing strategies can resolve this challenge and allow for more timely access to data to bring this level of context to always-on campaigns in near real-time. Additionally, using a single tool for audiences across channels helps ensure a fully coordinated experience for shoppers as they move from one channel to the next throughout their path to purchase.
Are You Ready to Optimize Paid Media Campaign Spend?
Paid media has proven to be something of a catch-22 for retailers: It holds so much potential to engage customers but can very quickly put a strain on budgets. However, it is possible to effectively optimize paid media spend, and it all starts with adding more intelligence to the audiences your team targets through these campaigns.
Running more intelligent paid media campaigns based on context like products of interest, purchase history, lifetime value and likelihood to convert can optimize ad spend by ensuring your team allocates resources to more effective campaigns (i.e. those that acquire and convert higher value customers and those that target customers who are actually ready to buy). Furthermore, it can not only help you target the best customers, but also engage them with the most attractive products and offers based on their areas of interest.
Visit bluecore.com/advertise to learn more about how you can benefit from our AI-powered audiences today.
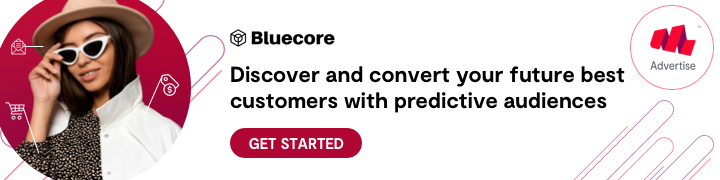